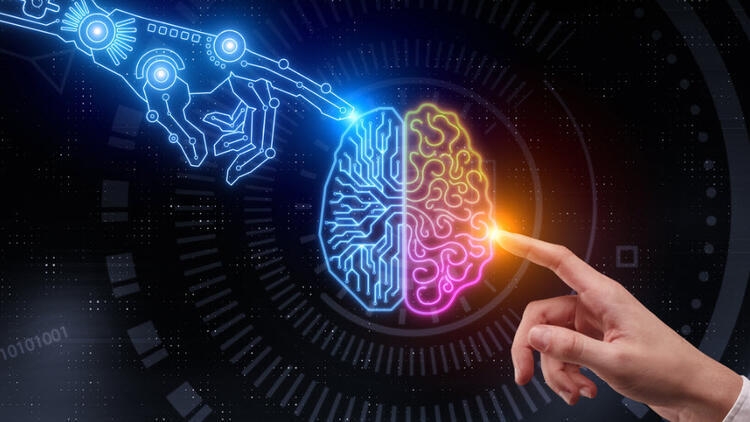
Can Supply Chains Run On Artificial Intelligence?
Uncategorized April 15, 2020, Comments OffSupply chains can be complicated ecosystems that may benefit from the application of artificial intelligence. In this article, we explore the possibilities of using machine learning technologies to automate several processes in the supply chain, possibly leading to beneficial monetary and non-monetary outcomes.
What Is Artificial Intelligence?
The term Artificial Intelligence (AI), has a non-standard definition that has evolved over time. Within academic circles, different schools of thought exist around the question of when an algorithm can be considered to be intelligent. Some purist may insist that AI can only be applied for technologies that can convincingly pass the Turing test. However outside of academic literature the common person usually considers any level of automated decision making to fit the definition of AI. This is regardless of whether the algorithm is itself capable of machine learning or not. It is that lay person’s definition of AI that we consider in this article.
What Is Supply Chain?
Supply chains now encompass several activities in addition to the movement of freight. Supply planning, demand projection, regulatory compliance, freight movement, warehouse management, vendor management and customer service can all come under the ambit of supply chain, depending which industry expert you choose to ask.
A.I In S&Op
It has become trendy for big multinationals to engage in a formal Sales & Operations Planning (S&OP) process to better manage inventory levels in readiness to meet demand changes. However, aligning demand and supply in most cases, is only accurate in the short term, with variance increasing exponentially over the length of time. This requires S&OP to be regularly recalibrated, sometimes even with a weekly frequency. There is no doubt that the decisions made during the planning cycle can be automated to a significant extent. Even without deep machine learning capabilities, statistical inputs from historical transactions can provide a powerful reference point for algorithms to draw conclusions on when to place supply requests, from who and for which customer.
A.I In Supply Route Determination
The true cost of transport to the shipper is always changing due to FX adjustments, changes in oil prices and changes in route fares. Coupled with unpredictable port closures, ocean conditions, port congestions, natural disasters and availability of connecting transports in multi-modal freight, it becomes almost impossible for the shipper to determine the most cost-efficient route to opt for on each shipment. These inputs, if fed into a system can be quickly translated into a supply route selection based on the shipper’s required bias towards cost, lead time or balance of both.
A.I In Hs Classification
Without extensive machine learning capabilities, it would be impossible for any technology to classify HS codes for any and all commodities that get traded across borders. However, when companies trade in only a specific range or type of product, the possibility for automated HS classification solutions exists. Currently, the process of HS classification requires a trained specialist who is familiar with the extensive text published by the World Customs Organization (WCO) on the subject. However, with even a simple multi-level binary type algorithm, a code can be determined based on a user’s inputs as long as the user is familiar with the product.
A.I In Warehouse Management
The use of AI in warehouse management is a big opportunity even for small warehouse operators. With basic machine learning capabilities, warehouse inventory management systems (WMS) can make optimized decisions concerning storage location allocations for products depending on expected flow through rates; placing high volume products on lower shelves for faster picking and slow-moving bulk items on higher racks so they remain out of the way until needed. An intelligent WMS may also be able to schedule redressing activities to occur during lull periods in warehouse operations, evening out the workload for employees.
A.I In Financial Compliance: Incoterms Cost Allocation
Using artificial intelligence, decisions can be made regarding the most beneficial international commercial trade terms (Incoterms®)to use between buyer and seller that maximizes cost efficiency for both shipper and consignee. Such a decision would be taken after considering various monetary factors such a cost of transportation and non-monetary factors such as clearance lead-times achievable by logistics providers available to both parties respectively.
What’s Stopping The Use Of Ai In Supply Chains?
AI is data driven and in order to derive an accurate outcome, an immense amount of data is required. When companies explore ways to use AI to make decisions that could seriously affect their top or bottom lines, they expect simulation outcomes to be significantly better than human results. However, the data required to support robust decision making is not always available and hence test scenarios may fall short of expectations, leaving corporations more comfortable with existing processes and unwilling to make the leap into automation.
In order to build the database of information required to support an accurate AI solution, companies will have to invest in resources to collect the important data-points accurately at scale. This additional cost is hence incurred even before costs of an AI solution can be explored.
This will remain a significant hurdle that obstructs the adoption of AI in supply chains, as today’s modern supply chains are driven on low margins, leaving little funding left to experiment on new projects that may or may not result in increased cost efficiency. Even fully automated warehouses that operate with a skeleton crew remain the novel exception rather than the norm, despite the obvious benefits of being able to run a warehouse with minimal human intervention.
As technological developments make the adoption of AI solutions less costly, more supply chains should be expected to look towards automated or AI driven solutions to cut costs, increase efficiency and improve flow through predictability and performance. However, it is likely that traction will be slow and solutions will not be of enterprise level, because as mentioned earlier, the data required to implement large scale AI systems do not yet exist and will first have to be collected over a substantial period of time.
References
https://www.tbmcg.com/resources/blog/6-ways-to-make-your-s-and-op-process-better/